Generative AI Governance on Amazon SageMaker | Amazon Web Services
If you’re delving into the world of artificial intelligence and looking to ensure governance and control over your AI projects, Amazon SageMaker might just be the tool for you. In this informative video shared by What’s New with AWS on Twitter, you can learn how to utilise Amazon SageMaker to safeguard and run governance controls for your end-to-end generative AI project.
Generative AI is a powerful technology that allows AI systems to create new content, such as images, text, or even music, based on patterns and data it has been trained on. With this incredible capability comes the need for robust governance to ensure ethical and responsible use of AI.
You may also like to watch : Who Is Kamala Harris? Biography - Parents - Husband - Sister - Career - Indian - Jamaican Heritage
Through Amazon SageMaker, AWS provides a platform that not only enables you to build and train your generative AI models but also offers tools for monitoring and managing these models throughout their lifecycle. This includes setting up governance controls to regulate access, monitor performance, and ensure compliance with regulatory standards.
By leveraging Amazon SageMaker for your generative AI projects, you can not only unleash the creative potential of AI but also do so in a responsible and controlled manner. So, if you’re keen on exploring the possibilities of generative AI while maintaining governance and control, dive into the video to learn more about how Amazon SageMaker can help you on your AI journey.
Generative #AI Governance on Amazon #SageMaker | #Amazon Web Services
In this video, you will learn how to use Amazon #SageMaker to safeguard and run governance controls for your end-to-end generative #AI project.
You may also like to watch: Is US-NATO Prepared For A Potential Nuclear War With Russia - China And North Korea?
Learn more at: https… #AWS https://t.co/MXTNQNVanX
— What’s New with AWS (@WhatsNewWithAWS) July 24, 2024
When it comes to utilizing generative AI on Amazon SageMaker, governance and control are crucial aspects to consider. In this article, we will delve into the importance of generative AI governance on Amazon SageMaker, exploring how you can safeguard your project and ensure that it runs smoothly. Let’s break down the key points step by step to give you a comprehensive understanding of this topic.
How can you ensure governance controls for your generative AI project on Amazon SageMaker?
Generative AI refers to AI systems that have the ability to create new content, such as images, text, or music, based on patterns and examples they have been trained on. While generative AI can be a powerful tool for innovation and creativity, it also comes with potential risks and challenges, especially in terms of ethics, bias, and privacy.
Why is governance important for generative AI projects?
Governance in the context of AI refers to the processes, policies, and controls put in place to ensure that AI systems are developed, deployed, and used responsibly and ethically. In the case of generative AI projects, governance is essential to mitigate risks such as bias in the generated content, infringement of intellectual property rights, and potential harm to individuals or society.
What are some key governance controls for generative AI projects on Amazon SageMaker?
- Data Governance: Proper data governance is essential to ensure that the training data used for generative AI models is accurate, representative, and ethically sourced. This includes implementing data quality checks, data lineage tracking, and data access controls.
- Model Governance: Model governance involves establishing processes for model development, validation, deployment, and monitoring. This includes version control, model performance tracking, and model explainability to ensure transparency and accountability.
- Ethical AI Principles: Amazon SageMaker provides tools and frameworks to help developers implement ethical AI principles, such as fairness, transparency, accountability, and privacy. It is important to consider the ethical implications of your generative AI project and take steps to address any potential biases or unintended consequences.
How can Amazon SageMaker help with generative AI governance?
Amazon SageMaker is a comprehensive machine learning platform that provides tools and services to streamline the development and deployment of AI models. With SageMaker, you can leverage pre-built algorithms, automated machine learning, and model monitoring capabilities to accelerate your generative AI project while ensuring governance and compliance.
What are some best practices for implementing governance controls on Amazon SageMaker?
- Establish Clear Policies and Procedures: Define clear policies and procedures for data governance, model governance, and ethical AI principles. Document these policies and communicate them to all stakeholders involved in the project.
- Implement Monitoring and Auditing: Set up monitoring and auditing mechanisms to track the performance of your generative AI models in real-time. This includes monitoring data inputs, model outputs, and user interactions to detect any anomalies or deviations from expected behavior.
- Regularly Review and Update Governance Controls: Regularly review and update your governance controls to adapt to changing requirements, regulations, and best practices in the field of AI ethics and governance. Stay informed about emerging trends and technologies that may impact your generative AI project.
In conclusion, generative AI governance on Amazon SageMaker is essential to ensure the responsible and ethical development of AI models. By implementing robust governance controls, you can safeguard your project and mitigate risks while unlocking the full potential of generative AI for innovation and creativity.
Source: Amazon Web Services
Remember, when working with generative AI on Amazon SageMaker, governance is not just a nice-to-have – it’s a must-have. So, take the time to implement the necessary controls and processes to ensure that your generative AI project is a success.
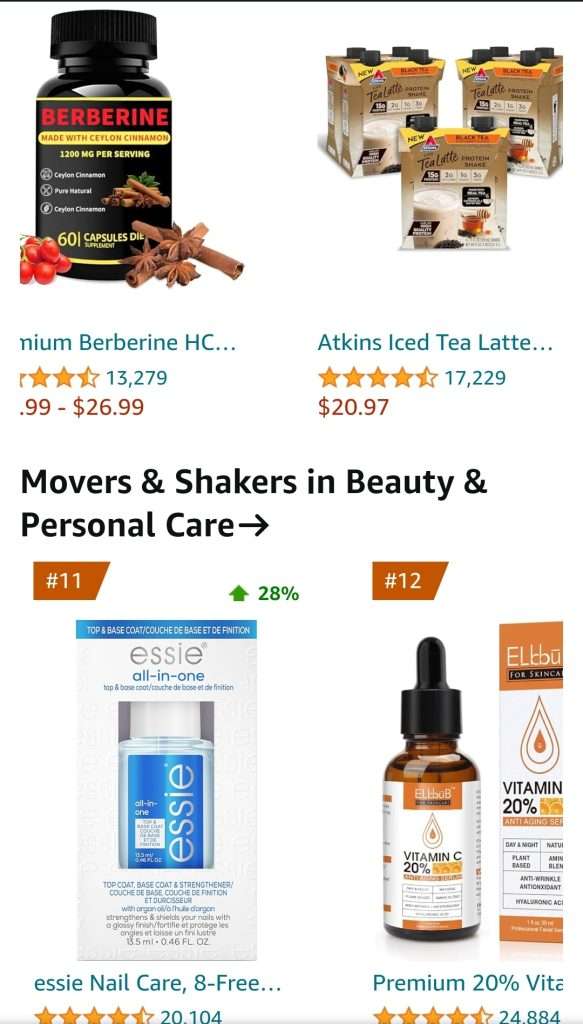
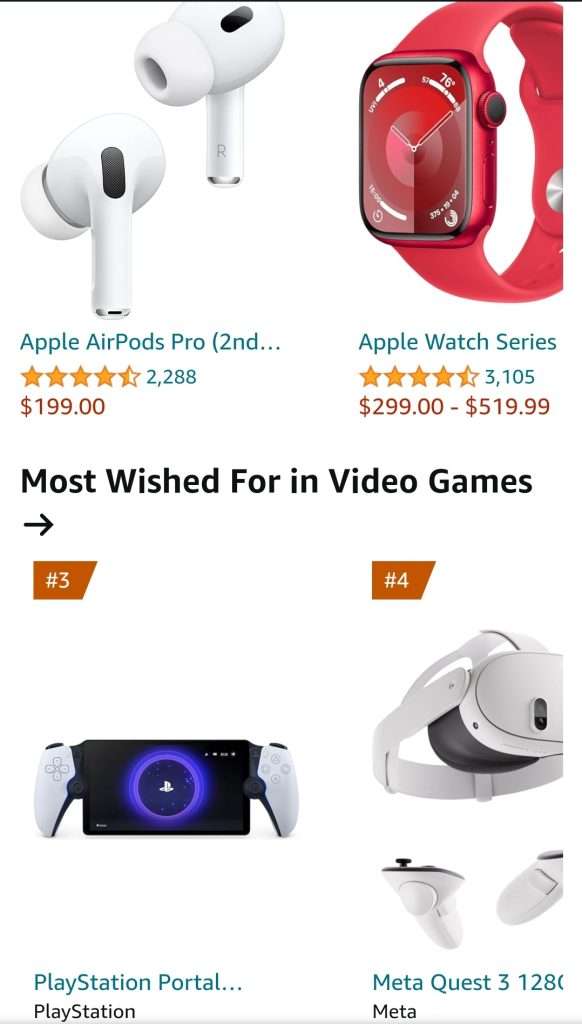
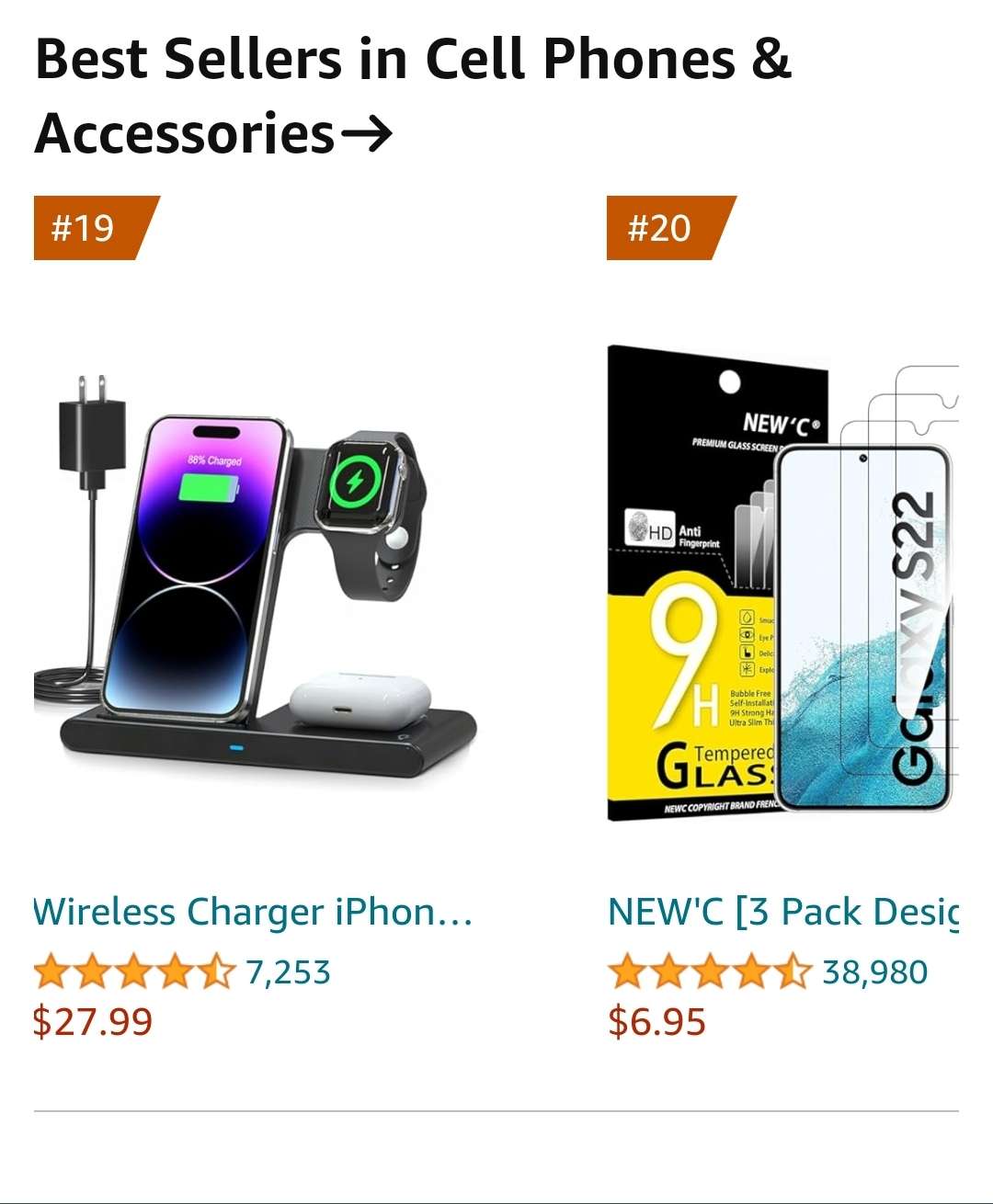