Breaking News: Beyond Binary Classification — Understanding Multiple Logistic Regression
In the world of data science, binary classification has long been the go-to method for predicting outcomes. However, there is a new kid on the block that is gaining popularity – multiple logistic regression. In this article, we will break down the basics of multiple logistic regression and explore how it goes beyond binary classification.
You may also like to watch : Who Is Kamala Harris? Biography - Parents - Husband - Sister - Career - Indian - Jamaican Heritage
Multiple logistic regression is a powerful statistical technique used to predict the probability of a categorical outcome with more than two possible values. It builds upon the principles of binary logistic regression but expands the scope to handle multiple categories or classes.
With the rise of big data and complex problems, the need for a more nuanced approach to classification has become apparent. Multiple logistic regression allows us to analyze data with multiple dependent variables, providing a more comprehensive understanding of the underlying relationships.
One of the key advantages of multiple logistic regression is its ability to handle categorical predictors. Unlike binary logistic regression, which requires dichotomous predictors, multiple logistic regression can accommodate variables with three or more categories. This flexibility enables us to incorporate a wider range of factors into our predictive models, leading to more accurate and robust predictions.
To illustrate the power of multiple logistic regression, let’s consider a practical example. Imagine a marketing campaign aimed at increasing sales in a retail store. The outcome variable could be categorized into “low,” “medium,” and “high” sales. By leveraging multiple logistic regression, we can analyze various predictors, such as advertising expenditure, store location, and customer demographics, to determine their impact on sales levels. This information can then be used to optimize marketing strategies and drive revenue growth.
You may also like to watch: Is US-NATO Prepared For A Potential Nuclear War With Russia - China And North Korea?
Implementing multiple logistic regression involves estimating the regression coefficients for each predictor variable. These coefficients represent the relationship between the predictor and the logit transformation of the outcome variable. By exponentiating these coefficients, we can interpret them as odds ratios, which provide insights into the relative likelihood of each category occurring.
It is important to note that multiple logistic regression assumes that the relationship between predictors and the outcome variable is linear on the logit scale. However, this assumption can be relaxed by incorporating higher-order terms or interaction effects into the model. These extensions allow for more complex relationships to be captured, enhancing the predictive capabilities of multiple logistic regression.
In conclusion, multiple logistic regression offers a more sophisticated approach to classification problems than traditional binary logistic regression. Its ability to handle multiple categories and incorporate categorical predictors makes it a valuable tool in the field of data science. By breaking down the basics of multiple logistic regression, we hope to inspire further exploration and application of this powerful technique.
If you are interested in learning more about multiple logistic regression and its applications, make sure to follow @rfeers on Twitter. Stay updated with the latest advancements in data science and unlock new possibilities in predictive modeling.
Remember, the world of data science is constantly evolving, and staying ahead of the curve is crucial. Embrace the power of multiple logistic regression and take your predictive modeling to new heights!.
Beyond Binary Classification — Breaking down Multiple Logistic Regression to its basics by @rfeers https://t.co/ShrqTFy2Vk
— Towards Data Science (@TDataScience) February 11, 2024
https://platform.twitter.com/widgets.js
Source
@TDataScience said Beyond Binary Classification — Breaking down Multiple Logistic Regression to its basics by @rfeers
RELATED STORY.
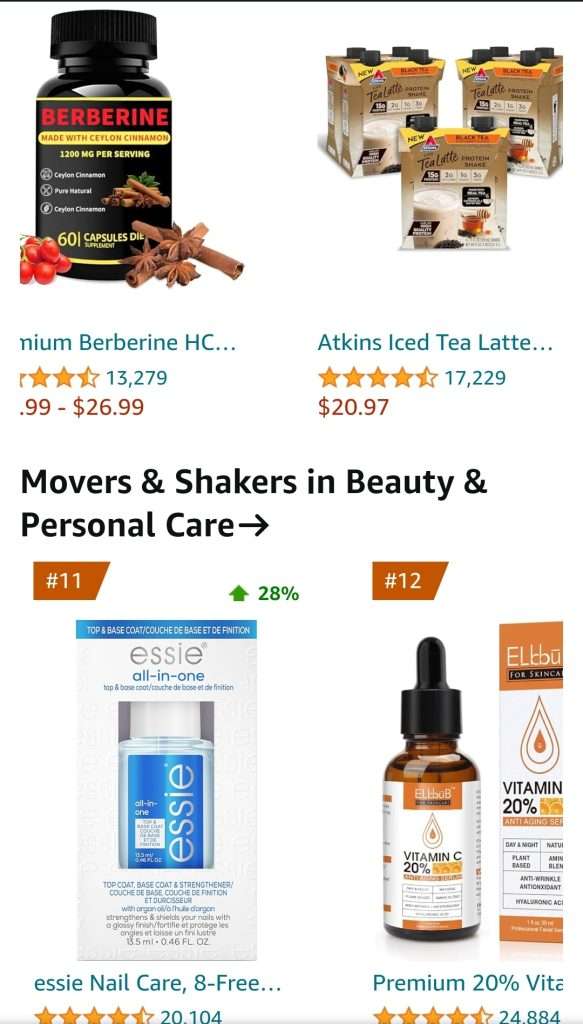
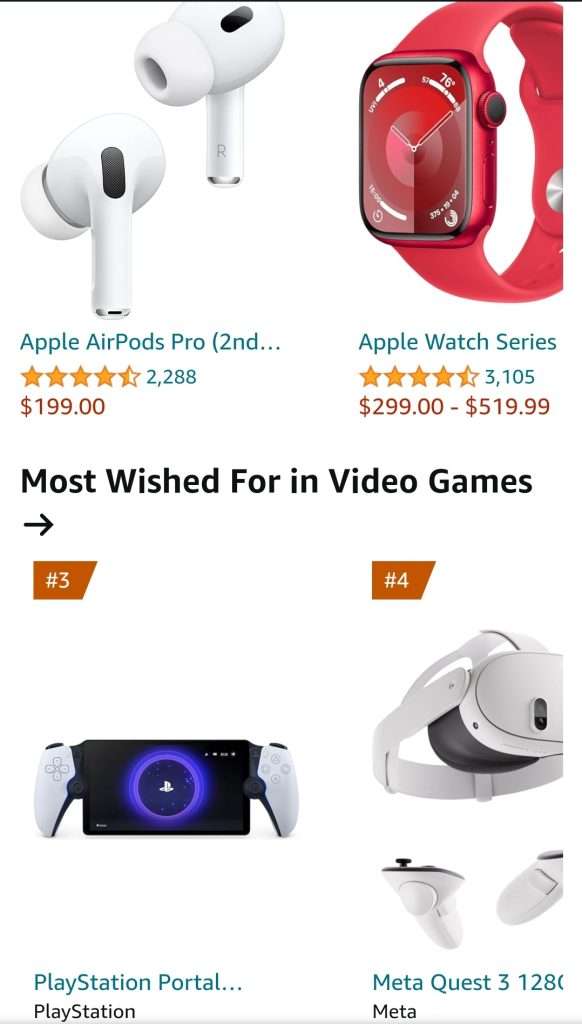
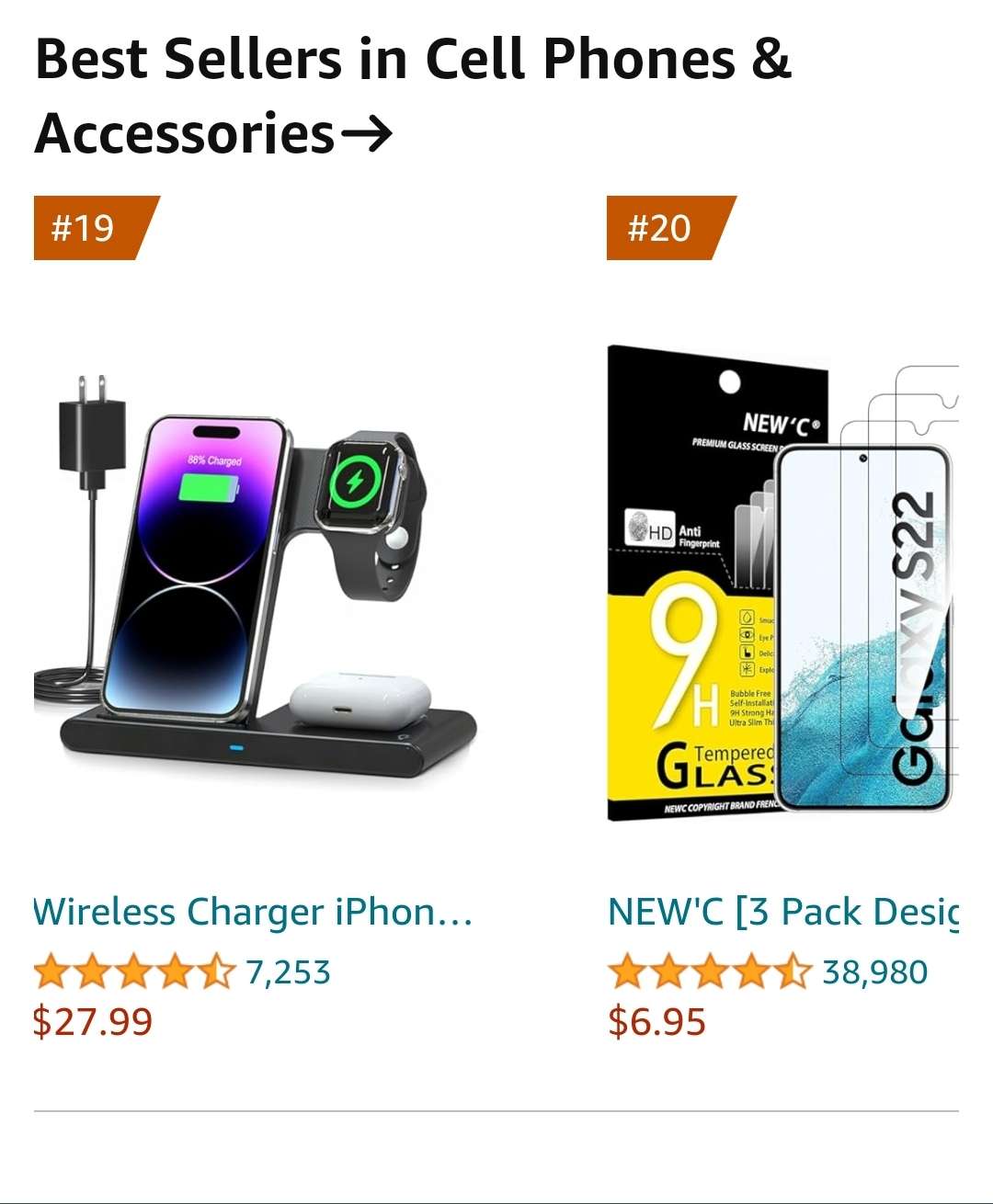